Editor’s note: When you implement a big data solution, choosing the right storage is the first order of business. Read on to learn about the big data solution options and don’t hesitate to explore our approach delivering to big data services, if you need to back up your big data project.
When ScienceSoft’s clients need to design their big data solution, we offer them to structure it with two storage elements: a data lake and a big data warehouse, which we distinguish from a traditional enterprise data warehouse. Here, looking ahead, we should say that a big data warehouse, unlike a data lake, is an obligatory element of a full-scale big data analytical solution. But, first things first, let us show you how data lakes and big data warehouses are different from each other in terms of architecture and their functional purpose.
The differences between a data lake and a data warehouse
Data state
ScienceSoft’s big data experts employ data lakes for storing all kinds of data – structured, unstructured and semi-structured. As for a big data warehouse, we use it as a storage for structured data.
The approach to storing data
A big data warehouse stores data according to the schema-on-write approach: before loading into the big data warehouse, data needs to be transformed into a unified structure to be fit for the big data warehouse.
A data lake stores data according to the schema-on-read approach: raw data is loaded into the data lake as it is and applied to the schema only when it is read. Thus, storing data in a data lake requires less effort.
Architecture
When speaking of a data lake, its flexible architecture may involve three elements:
- A landing zone – a transient area, where data undergoes preliminary filtering.
- A staging zone – a storage repository.
- An analytics sandbox – the area where data analysts perform experiments for exploratory data analytics.
When developing a data lake solution, our experts consider the staging zone the only obligatory element. If you want to learn more about the data lake zones and why we consider the landing zone and the analytical sandbox optional, study this article, here our data analytics researcher, Irene Mikhailouskaya, dwells on the data lake architecture.
As concerns the big data warehouse, it has a rigid architecture. Its elements are highly structured and obligatory as they are tied to business processes for the big data warehouse to correctly analyze and report data.
Storage costs
Drawing on our experience in rendering big data services, we have to admit that storing data in a big data warehouse is costly as you cannot load data unless it is of the required structure. And such a preparatory process is rather time- and resource-consuming. Thereby, we usually recommend our clients to consider integrating a data lake into the big data warehouse architecture as a cost-effective alternative: storing data in the data lake involves minimum or no data structuring before being loaded.
Users
Big data warehouses cater to the needs of business users and data analysts who use big data strategically to improve the decision-making process. Data lakes are mainly used as temporary storage of big data and the zone for data scientists and analysts to drive experiments.
Technologies
As both the big data warehouse and the data lake deal with big data, there is no difference in the technology stack to employ for storing, streaming and processing data:

Security
The use of big data is associated with certain security challenges. When developing big data solutions, ScienceSoft’s experts pay special attention to the high granularity of access control, when users’ access is limited depending on their roles. This measure prevents sensitive data leakage.
As opposed to big data warehouses, data lakes lack security focus due to the nature of stored data and its functional purpose. As only a limited number of users are granted the access, a data lake is protected as a whole, following the “all-or-nothing” approach.
The synergy of the data lake and the big data warehouse
Many big data project sponsors we talk to wonder if they can use a data lake or a big data warehouse alone in a data analytics solution. Our answer is it’s not an either-or choice: a data lake alone is never enough to design a full-scale big data analytics solution. We often recommend having the synergy of both. This is the case of businesses who need to both store large amounts of raw data to conduct experiments, and deliver intelligence to decision-makers. One of the telling examples when both elements function in sync within the one big data solution is an IoT solution, where the initial sensor data is stored in its raw format in the data lake, and then it undergoes the ETL\ELT process to be stored in the big data warehouse for further analysis. Such an alliance allows leveraging big data potential time- and cost-effectively.
How to start your big data journey?
Now, that you know your options, you need to decide whether your big data solution’s architecture will involve a big data warehouse and a data lake, or just a big data warehouse. To choose which way to go, you need to define:
- For what purposes your data will be used.
- What your requirements to data quality, speed of the data flow and the need for analytical experiments are.
- Who will use the data.
There are many factors to take into account and balance when deciding on the high-level big data architecture. We saw how long-drawn-out architectural decisions delayed actual big data implementation for years. And, unfortunately, we’ve witnessed how a wrong decision may result in massive rework later. ScienceSoft’s big data team would be happy to help with consulting or architecture design.
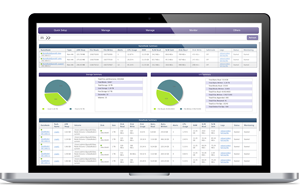
Big data is another step to your business success. We will help you to adopt an advanced approach to big data to unleash its full potential.