Editor’s note: In the article, Alex Bekker, Head of Data Analytics Department at ScienceSoft, explains how big data analytics can help a company drive revenue and reduce operational costs. Read on to learn how to start your big data journey and be welcome to explore ScienceSoft’s offer in big data services to learn what approach we follow to help our clients embrace big data potential.
As Head of Data Analytics, I enjoy studying the experiences of renowned companies who drive great value from big data initiatives, so that my team can offer our clients similar and even better results. Here, I’ve selected impressive big data use cases from the manufacturing industry, including, from ScienceSoft’s practice, that I hope will inspire you to embark on a big data journey.
#1. Production optimization
Extracting process improvement
A vertically integrated precious-metal manufacturer’s ore grade declined. The only logical way to avoid loss was to improve metal extracting and refining processes. Using sensor data, the manufacturer’s big data solution identified what factors influenced output the most. And the dominant parameter turned out to be oxygen level. With this insight, the team slightly changed the leaching process and increased the yield by 3.7%. Thanks to big data analysis, the manufacturer now earns $10-20 million additionally every year. Quite a gain, considering the ore grade deterioration rate was 20%.
Chemical yield perfection
A leading European chemicals manufacturer sought to improve yield. Using sensors, their big data solution analyzed how each input factor influenced production output. It analyzed temperatures, quantities, carbon dioxide flow and coolant pressures and compared their influence rates to one another. As a result, they revealed that carbon dioxide flow rates hugely affect the yield. And by slightly changing the parameters, they achieved a significant decrease in raw materials waste (by 20%) and energy costs (by 15%), and impressively improved the yield.
Vaccine yield improvement
A huge pharmaceutical company needed to find a way to improve the yield of their vaccines. To do that, the company’s big data solution analyzed their equipment sensor data, revealed interdependencies between various production parameters and compared how each of them affected the yield. Then, 9 most crucial parameters were identified, reviewed and adjusted to optimize the manufacturing process. It improved vaccines’ yield by 50%. Now, the company additionally makes $5-10 million a year per one substance.
Sugar-sweet optimization
High humidity levels and low-quality raw materials badly affected the taste of sugar of a large sugar manufacturer. To fight it, data science came in use to analyze sensor data and find correlations between the parameters contributing to the best sugar quality. Fortunately, with this insight the manufacturer managed to find a way to quickly influence product quality and achieve a unified sugar standard regardless of external factors. It allowed them to reduce production costs, increase customer satisfaction and simplify workloads.
#2. Quality assurance
Early-stage vehicle quality assurance
As early as 2014, BMW used big data to detect vulnerabilities in their new car prototypes. Data was collected from sensors on the tested prototypes and cars already in use. Due to big data analysis, BMW’s solution (probably integrated with their vehicle design and modelling software) spotted weaknesses and error patterns in the prototypes and in cars already in use. It enabled engineers to remove uncovered vulnerabilities before the prototypes actually went into production and helped reduce recalls of cars already in use. As a result, BMW can not only ensure higher quality at early stages, but also reduce warranty costs, boost brand reputation and probably save lives.
Jet engine design
Rolls-Royce uses big data extensively. And one of their most interesting manufacturing big data experiences is connected with modelling new aircraft engines. At the design stage, their software (integrated with a big data tool) creates simulations of new jet engines and analyzes terabytes of big data to see whether the new models are any good. This allows the company to find weaknesses before the model gets to production, which reduces defect-related costs and helps design the product of a much higher quality. Very smart, don’t you think?
#3. Enterprise management
Data-driven enterprise growth
Using big data analytics in manufacturing, companies can tackle global development challenges, such as transferring production to other countries or opening new factories in new locations. Companies’ historical and external data analysis can establish whether it’s still profitable to run factories in current locations or at current scopes by building predictive models and what-if scenarios. Besides, in the right hands, big data can help explore oceans of unseen opportunity, such as offering new products or even conquering new markets.
Accessible raw materials
To avoid costs connected with supply chain failures, an enterprise needed a better way to manage raw materials delivery. They decided to use their suppliers’ route details as well as weather and traffic data provided by trustworthy external sources to identify the probability of delivery delays. To do that, their big data tool (quite possibly integrated with their MRP) used predictive analytics and calculated possible delays and raw materials shortages. Based on these calculations, the enterprise worked out a supply-related emergency plan and is now able to run their production uninterrupted and avoid excessive downtime costs.
Predictive maintenance
Intel’s factory equipment live-streams IoT-generated data into their big data solution (probably integrated with MES). The analytics solution uses this data for pattern recognition, fault detection and visualization. It allows engineers to see what tendencies require their immediate attention and what actions are needed to prevent serious breakdowns on the shop floor. Such predictive maintenance reduces reaction time from 4 hours to 30 seconds and cuts costs. In 2017, thanks to big data and IoT, Intel predicted saving $100 million. This doesn’t look surprising at all: according to the research, predictive maintenance has appeared on companies’ radars exactly in 2017 and has got straight to top 3 big data use cases.
#4. After sales
Connected car vehicles
One of ScienceSoft’s customers from the connected car industry uses big data to provide after-sales support to their clients and ensure continuous improvement. The customer’s operational centers analyze in real time tons of data fed from car sensors (diagnostics data, mileage, geolocation, etc.) and generate insights into the product’s performance. The analysis of this data allows the company to monitor the product’s state, note and even predict some malfunctions and offer maintenance service early enough to avoid serious breakdowns. Such approach allows the customer to increase the product quality and enhance customer experience.
Hull cleaning
As a standard after-sales procedure, Caterpillar Marine was requested by one if their clients to do an analysis of how hull cleaning impacts fleet performance. Caterpillar’s big data solution (integrated with their Asset Intelligence platform) analyzed data from sensors on ships running with and without cleaned hulls. Then, it found correlations between the client’s hull-cleaning investments and fleet performance. Soon, Caterpillar concluded that their client needed to clean hulls more often (every 6.2 months, not 2 years) and that related investments paid off. As to the manufacturer, big data allowed them to ensure the most efficient exploitation of their products and improve the company’s image.
Wind farm optimization
As a proponent of after-sales with a personalized approach to customers in manufacturing, General Electric helps power producers use big data at 4 levels.
Level 1. Wind turbine’s sensor data analytics enables power producers to optimize turbine’s blade pitch and energy conversion automatically.
Level 2. Wind farm monitoring software compares sensor data to predicted values and recognizes performance patterns, which helps power producers perform preventive maintenance at the farms.
Level 3. Power producers use historical and real-time data to build predictive models, find correlations, detect faults and recognize patterns to optimize the farm’s work.
Level 4. The data is visualized and presented to top management for global-scale informed decision making.
A guide on how to start
If the examples of successful big data initiatives triggered your interest, I’ll gladly share a roadmap my colleagues at ScienceSoft and I devised for our clients to set off on a big data journey safely and effectively.
Ready… Set…
To prepare for a big data adoption project, the first thing crucial for success is finding the right approach. Rather than getting obsessed with the idea of big data, dashing to get the budget and then failing to extract value from it, first, you should lay the groundwork for the possible future ‘novelty.’ Let me show you the steps that will help you achieve business-IT alignment:
Step 1. I always advise big data project sponsors to start with reading about the possibilities of big data, then look at the business strategy and define what goals in it can be achieved with big data’s help.
Step 2. You should get more details on your company’s manufacturing problems and needs. The best way to do it is talking to the engineering management at your enterprise and asking them how the quality improvement process is going. Chances are, the process is problematic and no solution has yet been found, which is where you explain that such challenges can be solved with a thing called big data analytics.
Step 3. Try to get the consent of the engineering management to prove (if needed) to the company’s top management that they do need big data. And also warn them that their involvement will be necessary later to help data analysts understand the needed details of the manufacturing process.
Step 4. Determine a certain range of how much a particular big data project costs and talk to your top management about big data adoption and big data benefits. In our other article, my colleague, Olga Baturina, provided some telling statistics of big data gains.
If you need more details on how to ensure business IT-alignment, you can have a look at the guide written by my colleague, Boris Shiklo, CTO of ScienceSoft.
Go!
I always warn big data project sponsors against applying big data capabilities to complex tasks right from the start. Just like you can’t go to space a few days after deciding to become an astronaut. So, my advice to manufacturing companies is to start out with a simple project (for example, trying to achieve a stable output quality at a vaccine factory). A simple starting project allows you to see how big data can solve your problems with low risks and investments. Which, in its turn, is likely to positively affect your top management’s opinion on big data and encourage them to plan further big data investments (for more serious analytical projects).
At ScienceSoft, we usually break a big data project down into ‘digestible’ phases that are to be approached separately. Here are the sample phases of a big data project for manufacturing:
- Aggregating data.
- Using simple analytical algorithms.
- Turning to more sophisticated analytical methods.
- Incrementally automating your production management.
Aggregating data
Before any analysis can happen, you have to start aggregating data. In some cases, it’s not a problem at all: you just deploy/add sensors on your manufacturing equipment, prepare data storing facilities and enjoy the flow of ‘freshly-cut’ data.
In other cases, such as if your production cycle is months- or even years-long, it can prove difficult because you may lack the info on how your production process parameters influence output. And without knowing it, it’s all really a shot in the dark. But don’t get upset: there are ways to fight it. For example, in ScienceSoft’s projects, we recommend our clients to focus on one part of their manufacturing process, rather than on the entire process. That way they can improve the overall process by analyzing and adjusting its constituent parts.
Making analytical baby steps and advancing to big data strides
At ScienceSoft, we usually define the next stages of revealing big data insights:
- At first, you can perform relatively simple big data analysis to make targeted changes in your manufacturing processes (to improve product quality, for instance).
- Then, you can dig your data deeper to find ways to change your business processes. For example, you used to perform reactive maintenance and, with big data, you start preventive maintenance.
- When the time comes, you can even transform your business model, finding a better way to do it through big data analysis (say, you decide to get closer to the customer by making the cars you produce a smart connected product; you deploy sensors on them, analyze data from cars in use and provide after sales services).
The situation, I most commonly encounter, is that at early stages, customers only need the most usual analytical methods, such as correlations and regression analysis. As their big data competences and needs grow, analytical methods become more elaborate, and they start employing predictive analytics and machine learning in search of new business opportunities.
Production management automation
Automation of your production management is probably the most sophisticated way of using big data in manufacturing processes. The concept of automated production management is fairly simple: your historical and incoming sensor data is analyzed in real time and the control apps send targeted commands to actuators on your equipment.
A good example of production management automation is the case with General Electric’s wind turbines. Sensors provide data on energy generation and wind direction, according to which the blade pitch is changed to optimize the wind turbine’s efficiency.
An example to make it clear
Let me share an example of a generalized customer from my practice - a company who produces baby food and decides to go big data. They start with data aggregation (deploy/add data sensors on the production floor and prepare data storage).
For the sake of the example, let’s imagine that systematically, a few times a month, the baby food batches substantially drop in quality. Now, the big data team (together with the engineering team, R&D, product control managers) can find out what causes these quality drops. And together we realize that the manufacturing process doesn’t allow for the variations in the quality of raw material (baby food ingredients). If the ingredients’ quality is lower, the machinery isn’t ‘tuned’ to get a better quality output (say, you don’t adjust temperature and cooking times). And besides that, we also find a way to cut the production cycle duration. This big data application (better quality assurance) can be a good first project.
Getting valuable insights quickly and cheaply makes the company more interested in further big data capabilities and more complex analytical algorithms. And in a while, the enterprise starts running predictive analytics, equipment wear-out analysis and machine learning. Among other things, it allows them to perform predictive maintenance, which enables the staff to react to alarming trends on the manufacturing floor before any real damage is caused.
And as the company expands globally, we help the company to use big data powers to assure and control baby food quality across all the franchisees.
Now, survive
Yes, while starting big-data-adoption action, there are always impediments. Big data project sponsors I talk to commonly voice the following concerns:
- Lacking in-house technical skills.
I believe, not every business needs complete outsourcing. For example, at early stages, when you’ll need to experiment a lot, it’s simply easier, if your ‘domestic’ people are involved, thus it’s natural to hire new skilled tech employees or retrain old ones.
- Lacking the understanding of big data potential
In case of outsourcing a big data project, your vendor will need to work closely with your team (the engineering team, R&D, product control managers, etc.) to ensure the deep understanding of manufacturing processes. Together with a vendor who has a solid approach to cooperation, you’ll be able to see elaborate ways to improve production and its management with big data potential.
- Resisting the new technologies.
Some employees – let’s hope the lesser part – will probably resist big data. And there’s nothing personal about it: for creatures of habit, it’s just more convenient to use the old technologies. Training your staff as well as controlling their usage of the new solution can help deal with this challenge.
You are now ready
The manufacturing use cases show that big data can bring big money and big value. They also show that big data is most widely used for production optimization. And it’s quite logical: big data solutions are really good at finding correlations. While production changes based on sensibly selected correlations can improve yield enormously.
To reap the benefits that big data offers and start using big data in your manufacturing organization, you need to carefully plan your actions. So, let’s rehearse them. You should:
– Find the right approach to your big data. Carefully analyze your business needs, find a way to fulfill them with big data.
– Prudently plan your big data adoption. Don’t jump to the most difficult part right off the start. Find a small-scale project to test big data on. Aggregate data, test simple algorithms and then try more daring ones.
– Watch for management challenges. Gain a thorough big data understanding, don’t rush into outsourcing the project completely and engage a needed number of engineering technologists.
But before starting some real action, I advise you to turn to big data consulting, since it can ease the hardships of big data projects and contribute to big data understanding. If you want to know more about our big data consulting services, reach out to me.
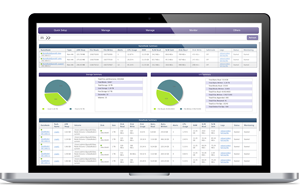
Big data is another step to your business success. We will help you to adopt an advanced approach to big data to unleash its full potential.