How to get several incredibly accurate computer vision algorithms? Create a database containing 12+ mln images and make scientists from around the globe compete to design and develop the best image analysis software. Worked well but you need even more precision? Repeat annually until satisfied (and don't forget about a cake for the winner!).
That was the recipe of the ImageNet Large Scale Visual Recognition Challenge (ILSVRC) a competition held every year by the collaboration from the University of North Carolina at Chapel Hill, Stanford University, and the University of Michigan.
Who they are
This all started back in 2010 with a group of Stanford researchers headed by the leading AI scientist Fei-Fei Li. They collected a database of labeled images comprehensively reflecting the real world around us there are animals, plants, various inanimate objects, different human activities, etc. "We were thinking about coming up with the dataset that is not a dataset that reflects the statistics of the web but rather an anthology that tabulates the visual knowledge in human understanding that was the philosophy of ImageNet and where it started", Fei-Fei says.
Indeed, big and comprehensive data is the key to successful machine learning, and, subsequently, to computer vision. The ImageNet database now contains 14,197,122 images classified into 17 thousand categories, and these are the training data for ImageNet Challenge. The test and validation datasets contain several hundred thousand images and up to a thousand categories depending on the task.
What they want
Each year, dozens of collaborations present their algorithms for ILSVRC, competing in object detection and classification precision. The ultimate goal is the detection and classification accuracy comparable to that of a human or even exceeding it.
And the latter isn't some farfetched speculation the algorithms designed by ILSVRC-2015-winning Microsoft Research Asia team already have shown a superior to human's ability in several particular image recognition tasks. To achieve this spectacular result, MSRA team has used deep residual nets (up to 152 layers) and the "Faster R-CNN" system, composed of a deep fully convolutional network that proposes regions and the Fast R-CNN detector that uses the proposed regions[1].
However, scientists emphasize that this is only a tiny part of a final computer vision solution. Human vision is much more complicated, as humans understand a scene as a whole, not some stand-alone objects, and are able to retrieve its context and to predict its development. Now, even more attention is paid to a relatively new ILSVRC category object detection from video. In particular, this task is very important for the self-driving car and drone industries.
When they want it
Looking at the results of the ILSVRC winners achieved in the last 4 years, we can see major leaps in object detection and classification both in 2014 and in 2015. However, the last year's moderate growth might indicate that the pace of development in the image classification is throttling down.
The results of ILSVRC 2017 will be released on July 5, 2017. We still don't know who are this year's participants and what algorithms they have up their sleeves, but we can expect one more leap forward, at least in the new object-detection-from-video task, as by now this is the challenge scientists hurl all their effort into.
Why we care about that
Computer vision is one of the flagships of today's software development. Besides above-mentioned self-driving cars and drones, this technology is widely used in manufacturing for automated visual inspection and in healthcare as a part of computer-aided diagnosis systems. We always stay tuned for new advances in object classification algorithms, looking for new great results that will raise the accuracy of computer vision. So, mark the 5th of July in your calendar to find out what news ImageNet Challenge 2017 is going to bring.
References:
- "Faster R-CNN: Towards Real-Time Object Detection with Region Proposal Networks", Shaoqing Ren, Kaiming He, Ross Girshick, Jian Sun. NIPS 2015.
That’s how we do it
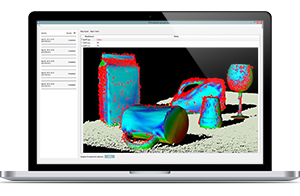
Image Analysis by ScienceSoft
Detect, recognize, identify. In a word, get meaningful info from your images and videos to solve countless business tasks.
That’s how we do it