If you think that with big data you’ll cast a spell and easily boost your business, take off your cloak and throw away your wand because big data isn’t magic. But if you roll up your sleeves and do some cleaning, that may do the trick and help you achieve a stunning business result.
Big data is indeed powerful but not too perfect. This article shows that it poses multiple challenges and data quality is one of them. Lots of enterprises recognize these issues and turn to big data services to handle them. But why exactly do they do that, if big data is never 100% accurate? And how good is good big data quality? That you will find out.
What if you use big data of bad quality?
Relatively low quality of your big data can be either extremely harmful or not that serious. Here’s an example. If your big data tool analyzes customer activity on your website, you would, of course, like to know the real state of things. And you will. But keeping 100%-accurate visitor activity records would not be necessary just to see the big picture. In fact, it wouldn’t even be achievable.
However, if your big data analytics monitors real-time data from, say, heart monitors in a hospital, 3%-margin of error may mean that you failed to save someone’s life.
So, everything here depends on a particular company. Sometimes even on a particular task. And this means that before rushing to push your data to the highest level of precision possible, you need to stop for a moment. First, you should analyze your big data quality needs and then establish how good your big data quality should be.
What exactly is good data quality?
To distinguish bad or dirty data from good or clean, we need a set of criteria to refer to. Although, you should note that these apply to data quality on the whole, without associations with big data exclusively.
There are a number of criteria sets when it comes to data quality, but we have selected 5 most important data characteristics that should ensure your data is clean.
- Consistency – logical relations
In correlated data sets, there should be no inconsistencies, such as duplications, contradictions, gaps. For instance, it should be impossible to have two similar IDs for two different employees or refer to a non-existent entry in another table. - Accuracy – the real state of things
Data should be precise, continuous and should reflect how things really are. All calculations based on such data show the true result. - Completeness – all needed elements
Your data probably consists of multiple elements. In this case, you need to have all the interdependent elements to ensure that the data can be interpreted in the right way. Example: you have lots of sensor data, but there’s no info about the exact sensor locations. This way, you won’t really be able to understand how your factory’s equipment ‘behaves’ and what influences this behavior. - Auditability – maintenance and control
Data itself and data management process on the whole should be organized in such a way that you can perform data quality audits regularly or on demand. This will help to ensure a higher level of data adequacy. - Orderliness – structure and format
Data should be organized in a particular order. It needs to comply with all your requirements concerning data format, its structure, range of adequate values, specific business rules and so on. For instance, the temperature in the oven has to be measured in Fahrenheit and can’t be -14 °F.
* If you’re having trouble remembering the criteria, here’s a rule that might help: all their first letters together make the word ‘cacao’.
Any difference with big data quality?
If speaking strictly about big data, we have to note: not all these criteria apply to big data and not all of them are 100%-achievable.
The issue with consistency is that big data’s specific characteristics allow for ‘noise’ in the first place. The huge volume and structure of big data make it difficult to delete all of it. Sometimes, it’s even unnecessary. However, logical relations within your big data have to be in place in some cases. For instance, if a bank’s big data tool detects potential fraud (say, your card was used in Cambodia while you live in Arizona). The big data tool monitors your social networks. And it can check whether you are on a vacation in Cambodia. In other words, it relates info about you from different data sets and, therefore, needs a certain level of consistency (an accurate link between your bank account and your social network accounts).
Whereas while collecting opinions on a particular product in social networks, duplications and contradictions will be acceptable. Some people may have multiple accounts and use them at different times, in the first case saying that they like the product and in the second – that they hate it. Why is it alright? Because on a large scale, it won’t affect your big data analytics results.
Concerning accuracy, we’ve already outlined earlier in the article that its level varies from task to task. Picture a situation: you need to analyze info from the previous month and data worth 2 days vanishes. Without this data, you can’t really calculate any accurate numbers. And if we’re talking about TV commercials’ views, it’s not that critical: we still can calculate monthly averages and trends without them. If, however, the situation is more serious and more sophisticated calculations or thoroughly detailed historical records are needed (like in case with a heart monitor), inaccurate data can lead to erroneous decisions and even more mistakes.
Completeness also isn’t a thing to worry about too much because big data naturally comes with a lot of gaps. But it’s OK. In the same case when the data from 2 days vanished, we still can get decent analysis results because of a huge volume of other similar data. The whole picture will still be adequate even without this measly part.
As to auditability, big data does provide opportunities for it. If you want to inspect your big data quality, you can. Although, your company will need time and resources for that. For instance, to create scripts that’ll check data quality and to run these scripts, which may be costly due to big data volumes.
And now to orderliness. You should probably be ready for a certain degree of ‘controllable chaos’ in your data. For instance, data lakes don’t usually pay much attention to data’s structure and value adequacy. They just store what they get. But before data is loaded into big data warehouses, it usually does undergo a cleansing procedure, which may partially ensure your data’s orderliness. But only partially.
Stay ‘dirty’ or go ‘clean’?
As you see, none of these big data quality criteria are strict or suitable for all cases. And tailoring your big data solution to satisfy all these to the fullest may:
- Cost a lot.
- Require lots of time.
- Scale down your system’s performance.
- Be quite impossible.
This is why some companies neither chase after the clean data, nor stay with the dirty one. They go with ‘good enough data’. It means they set a minimal satisfactory threshold that would give them adequate analytics results. And then they make sure their data quality is always above it.
How to improve big data quality?
We have 3 rules of thumb for you to follow when deciding on your big data quality policy and performing any other data quality management procedures:
Rule 1: Be cautious about data sources. You should have a particular hierarchy of reliability for data sources since not all of them bear equally decent information. Data from open or relatively unreliable sources should always be verified. A good example of such a questionable data source is a social network:
- It can be impossible to trace the time when a particular event mentioned on social media happened.
- You can’t be sure about the origins of the mentioned information.
- Or it can be difficult for algorithms to recognize emotions conveyed in user posts.
Rule 2: Organize proper storage and transformation. Your data lakes and data warehouses need to be looked after, if you want good data quality. And a fairly ‘strong’ data cleaning mechanism needs to be in place while your data gets transferred from a data lake into a big data warehouse. Besides that, at this point, your data needs to be matched with any other necessary records to achieve a certain level of consistency (if needed at all).
Rule 3: Hold regular audits. This one we’ve already covered, but it deserves extra attention. Data quality audits, as much as any audits of your big data solution, are an essential part of maintenance process. You may need both manual and automatic audits. For instance, you can analyze your data quality problems and write scripts that will run regularly and inspect your data quality problem areas. If you have no experience in such matters or if you’re unsure whether you have all the needed resources, you can consider outsourcing your data quality audits.
Got it?
Data quality issues are a complex big data problem. Here’s a shortcut to recap the main points:
Q: What if you use big data of bad quality?
A: It depends on your domain and task. It may influence you only slightly, if you don’t need high precision, but it can also be very dangerous, if your system needs extremely accurate data.
Q: What is good data quality?
A: There are 5 “cacao” criteria of big data quality. But they are not suitable for all. Every company has to decide what level of each criterion they need (on the whole and for particular tasks).
Q: How to improve big data quality?
A: Be cautious about data sources, organize proper storage and transformation and hold data quality audits.
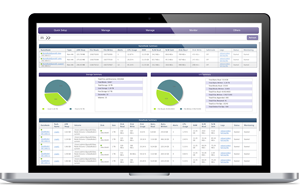
Big data is another step to your business success. We will help you to adopt an advanced approach to big data to unleash its full potential.