Editor’s note: Are you eager to go big data? Slow down and read on about the problems that may arise when developing a big data project. As a big data service provider, ScienceSoft can help you surmount these problems and leverage the big data potential to the fullest.
Big data presents not only multiple challenges, but also serious problems. And here is the difference between them.
If you are walking to a shop and a huge puddle appears in your way, you can try to walk past it or jump it over. If the puddle is too big, you can ask someone to help (turn to ‘puddle consulting’). Then, experts can drain the puddle. This is a challenge: an impediment in your way that can be solved relatively easily.
But later you realize that, to get to the shop, you need to walk through a bad neighborhood. And it would be too long a way to go around it because the shop can close before you arrive. This is a problem: a more fundamental issue that can cause trouble.
Obviously, these walk-related issues can’t compare to big data problems, but the concept is yet the same: challenges are on the surface, while problems are of deeper nature. In this article, we’ve already covered what big data challenges you can face. But what problems are there?
Problem #1 – Imperfect big data analytics
Although data scientists everywhere are trying their best to improve data quality and make analytical algorithms more robust (immune to data-related problems), big data analytics is not perfect. It is just not yet possible to solve some of the issues connected with your data’s reliability.
More ≠ better
Much like in a heavy snowfall, data is piling up at a high speed and in a gigantic volume. You could think that it’s good: more data means more reliable insights. But in reality, huge volumes of data don’t necessarily mean huge volumes of actionable insights. Sometimes, the data you have – despite all the info it contains – statistically just isn’t the representative sample of the data you need to analyze. For example, opinions on Twitter vs. opinions of the population on the whole. Let alone the bias of the former, it doesn’t even contain the viewpoints of the entire population, (for example, the elderly and the introverts often get excluded). This way, you can get wrong analysis results easily.
Besides that, in such ‘heavy snowfalls’, it simply gets more challenging to find what you need while eliminating the data that bears no use whatsoever.
Weird correlations
We all know that big data is good at finding correlations. If there are any, it’ll find them all. But the thing is that the correlations that big data finds sometimes aren’t meaningful at all. Suppose the overall number of AC/DC’s songs bought in the US throughout the year decreased and so did the US crime rate. Would it mean that AC/DC’s music incites people to break the law? No. But big data would show you this correlation anyway. And this is how you can waste lots of time, manually searching for truly meaningful correlations in the sea of weird.
Going in circles
If a text is machine-translated, say, from Urdu into Japanese, there is a huge chance that the result is at least a bit inaccurate in a few places. But if such a text is then left alone, it’s not too bad. It is much worse when such an inaccurate translation is used by another big data algorithm as a ‘source of truth’. The results of big data analysis will be far from adequate, if a big data tool uses as raw data the chunks of information generated by another big data algorithm. And the more ‘circles’ there are, the worse the outcome.
Sly users
Big data algorithms are often based on specific markers ‘attached’ to the analyzed item. And due to this, big data analytics results can be ‘falsified’. As soon as someone figures out what markers influence the outcome, they can adjust the analyzed items to satisfy the requirements set by the markers. The best illustration here would be sly students and their efforts to cheat points-scoring software.
‘Rares’ and ‘subjectives’
Not everything, by its nature, can be analyzed just by crunching the numbers. The more subjective or rare the analyzed item, the bigger the possibility for inadequate results. As an example of the ‘rares,’ let’s see how Google can translate a poem. The answer would be: “Very poorly.” Partially because poets tend to use exquisite and eloquent phrases that Google hasn’t ever seen. But it doesn’t mean that these phrases are incorrect or can be substituted by synonyms, does it?
And as an example of the ‘subjectives,’ let’s try to ask a big data analytics tool to tell us what poets are the most influential in history. There are different ways to get an answer here, but chances are, it won’t be too precise. And it’s understandable: in spite of numerous evident objective factors, such a question is deeply subjective.
Problem #2 – Hasty technological advancement
Techno-uncertainty
As far as we can see, there are no factors that can curtail the technological advancement of big data. It will evolve further and maybe even at a greater speed, which is exactly the problem. At such a pace, it’s difficult to foresee whether it will be efficient to solve your future challenges with the technology you have to choose today. Just like with a smartphone, you can buy the hottest one yet but in a year it will be old and not gold.
Still underqualified
One of the oldest problems with big data is the lack of qualified specialists in the field. It was so in 2014 and it is so in 2018. And the fast technological advancement also contributes to the cause. As a result, lots of companies have to retrain their own staff or deal with underqualified specialists from ‘outside’.
Problem #3 – Negative social impact
Big data is unlikely to influence society as much as the appearance of mobile phones, but it still causes some alarming trends that concern everyone.
‘D’ for discrimination
As stated above, big data analytics relies on certain markers of analyzed items. If the person attaching the marker is biased towards the issue, it will affect the result. Hence, biased markers equal biased analysis. And for some software, it is highly disturbing. If a bank credit-scoring app views your social networks and sees that you like rap music, you may score less and not get the much-needed loan. Essentially, this is just another way to discriminate people.
No more privacy
Suppose you go to a travel agency website to check how much it costs to go to Greece for the summer. Then, you make your way back to whatever important task you were doing. And while browsing through work-related info on the web, you suddenly start to see endless ads about Greece travel packages. Happens quite a lot, doesn’t it?
Big data mechanisms are used to spot your interest in a particular product or service and then make a personalized offer to boost sales. And as long as such targeted offers are relevant and don’t invade your ‘personal web space’, it’s OK.
But if you start to think about it, how many strangers now know that you will be spending time in Greece this summer? And while this example is rather harmless, what if your current location gets into the wrong hands?
For now, these questions remain unanswered. Governments in the US and Europe are trying to fight it, but uncontrolled use of our personal information still leaves little – if any – room for privacy on the Internet.
Don’t get depressed though
Despite all the big data problems, you shouldn’t get nervous and try to avoid big data as much as you can. Yes, it’s not yet possible to find a solution to all of them (imperfect big data analytics, privacy violation issues, hasty technological advancement). But with the help of experienced big data consulting experts, it is more than possible to find and implement certain workarounds. This way, big data problems will just be somewhere in the background, while your business thrives and ascends the stairway to heaven (on Earth).
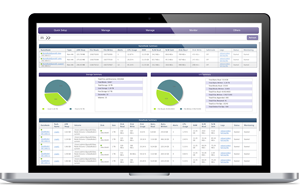
Big data is another step to your business success. We will help you to adopt an advanced approach to big data to unleash its full potential.