Having implemented a business intelligence solution long ago, you keep monitoring recent trends to understand what the market can offer. While doing your research, you must have noticed that business intelligence often goes side by side with data analytics. Does this mean that you can enrich your existing BI solution by following promising data analytics trends? Is the synergy of BI and DA possible? Let’s find this out.
Business intelligence and data analytics – two sides of the same coin
A quick search on the internet is enough to understand that these two terms are used inconsistently: different vendors, service providers and other market players keep to their internal definitions. That is why, some sources consider business intelligence and data analytics two different concepts, while the others use these terms interchangeably. We define them as follows:
Business intelligence (BI) is a technology-based process of analyzing data and presenting actionable insights to help business users make informed decisions. The implementation of BI includes three main stages:
- Developing a data warehouse
- Designing online analytical processing (OLAP) cubes
- Visualizing data.
Data analytics is a catchall term that encompasses business intelligence as well as advanced approaches and methods to collecting, processing and analyzing data sets to identify trends, dependencies and correlations. The term is broad and is applicable to both business and science. Data analytics includes:
- Data mining
- Predictive and prescriptive analytics
- Big data analytics, etc.
How to achieve the synergy of BI and DA
Usually invisible to end business users, data analytics uses complicated algorithms and statistical approaches to provide extra insights, which then can enrich habitual reports. Here, we share some illustrative examples of how business intelligence and data analytics can work together.
- Cohort analysis allows considering online store visitors not as a whole, but broken down into different user groups that show similar behavior patterns. Such groups may become a dimension for the OLAP cube. Business decision makers can compare them by sales, profit, the number of orders per month, etc. to design personalized marketing activities.
- Regression analysis allows identifying the relationship between variables. The dependency (or the lack of dependency) between them can provide companies with extra insights, as opposed to historical data alone. For instance, it is interesting to look at the total number of complaints and top-10 complaints. But with regression analysis, you may also find out whether the wait time and the number of complaints are connected.
- Time series analysis is applied to historical data to create forecasts. Let’s say, you want to predict sales. For this, you need to have sales figures for several previous years, split by month. Based on this data, an analytical system will identify past trends, monthly growth/decline rates, repeating patterns, if any, and will make the best possible estimate for the future.
Data analytics trends are definitely worth attention
Let’s look at some data analytics trends and find out how they can help enrich your existing BI solution. By the way, you may find the same trend in both BI and data analytics lists (do you remember the inconsistency of the terms we mentioned above'). The implementation of some initiatives will cause little pain, while others may require significant changes in the technology stack, approaches and methods.
1. Machine-learning based artificial intelligence
Let’s talk here about the problem of customer churn. Traditional BI solutions help you understand how many customers left you last week/quarter/month. Looking at the churn rates, you naturally start thinking about how to return these customers. Still, the moment is gone – the customers have already switched to your competitors, and now you’ll have to do your best to win them back.
With machine learning-based AI, businesses can identify high-risk customer segments well in advance. The analytical system can assess customers’ activities across all channels and signal if their behavior looks like they are going to leave. For example, a customer contacts the support center more frequently than the average customer does, or they start using your services less often, or their average spend significantly decreases. Of course, the set of symptoms will be specific to each industry. And you need to identify the ones that are essential for your business, score each symptom and let your analytical system learn. As a result, your system will inform you about a possible churn well in advance for you to take actions, such as targeted marketing campaigns.
2. Predictive analytics
Having timely and accurate reports that depict historical data is great. However, many businesses may find this insufficient. In fact, companies also need to understand what is likely to happen in the future to take preventive actions today. Here, predictive analytics comes to rescue.
Imagine a manufacturer of outdoor clothing who is planning their range for the next winter season. They may look at past sales split by category and plan production volumes accordingly. But will it be insightful? Alternatively, they may apply a time series analysis that we have described above.
As fashion is fast-paced, the manufacturer needs even more insights to forecast customer demand, decide on the winter range and plan the production volume for every item. For example, the producer can additionally analyze weather forecasts (the colder the winter, the less three-quarter coats should be in the range) and the trends that are becoming popular on social media.
3. Big data
If your business is on the brink of an important change that will require collecting, processing and analyzing big data (such as installing sensors to your machinery to foster preventive maintenance or launching an e-store in addition to brick-and-mortar ones, etc.), your analytical system should also be able to handle this new challenge. A traditional BI solution should be extended. Big data requires a dedicated technology stack, such as Apache Hadoop, Apache Hive, Apache Spark, etc. To get valuable insights from big data sets, you have a wide variety of data analytics methods and techniques at your disposal, for instance, pattern matching.
On a final note
If you have a BI solution implemented, this does not mean that you have hit the ceiling. The market is always developing and offering new business intelligence services. There are always ways to improve the existing solution.
While checking the recent trends, don’t limit yourselves to business intelligence only. Check out the list available for data analytics, as well. For some businesses, traditional business intelligence may suffice, but some companies may find it reasonable to enrich the existing solution with data analytics to get more insights.
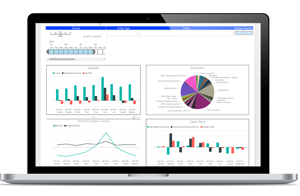
Are you striving for informed decision-making? We will convert your historical and real-time data into actionable insights and set up forecasting.